2016 International Conference on Intelligent
Computation
August 2-5,2016
Lanzhou,China
(
http://www.ic-icc.cn/2016/index.htm
)
![]() |
Vladimir Cherkassky IEEE Fellow University of Minnesota, USA |
![]() |
Frank L. Lewis National Academy of Inventors, Fellow IEEE, InstMC, and IFAC University of Texas at Arlington, USA |
![]() |
Asoke K.Nandi FREng, FIEEE, FIET, FIMA, FInstP, FRSA, FIMechE, FBCS Brunel University London, UK |
![]() |
DeLiang Wang IEEE Fellow Ohio State University, USA |
![]() |
David Zhang IEEE FIAPR Polytechnic University, Hong Kong |
Reliable Prediction of Epileptic Seizures from EEG Signal
Vladimir Cherkassky
Electrical & Computer
Engineering
University of Minnesota
Minneapolis MN 55455
Email: cherk001@umn.edu
http://www.ece.umn.edu/users/cherkass/predictive_learning/
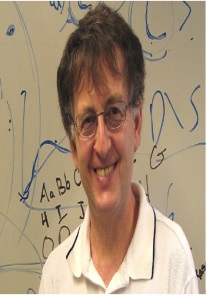
Abstract: there is a growing interest in data-analytic modeling for prediction and/or detection of epileptic seizures from EEG recording of brain activity. In spite of evidence that many patients have changes in EEG signal prior to seizures, development of robust predictive methods remains elusive. We argue that the main obstacle for development of effective EEG-based predictive models is an apparent disconnect between clinical and data-analytic modeling assumptions and constraints. We present an SVM-based system for reliable seizure prediction, where design choices and performance metrics are clearly related to clinical objectives. This system achieves very accurate classification / discrimination between preictal and interictal states in dogs with naturally occurring epilepsy. We argue that sound application of machine learning methods for predictive modeling requires proper formalization of clinical objectives in the context of statistical assumptions underlying existing machine learning algorithms. In this respect, the main promise of Big_Data (i.e., more data more knowledge) appears counterproductive, as it ignores the role of human intelligence and good engineering, which cannot be outsourced to statistical or machine learning software.
Bio-Sketch: Vladimir Cherkassky is Professor of
Electrical and Computer Engineering at the University of
Minnesota, Twin Cities. He received his PhD in Electrical
and Computer Engineering from the University of Texas at
Austin in 1985. He has worked on theory and applications of
statistical learning since late 1980’s and he has
co-authored the monograph Learning from Data,
Wiley-Interscience, now in its second edition. He is also
the author of a new textbook Predictive Learning - see www.VCtextbook.com
He has served on editorial boards
of IEEE Transactions on Neural Networks (TNN), Neural
Networks, Natural Computing, and Neural Processing Letters.
He was a Guest Editor of the IEEE TNN Special Issue on VC
Learning Theory and Its Applications published in September
1999. Dr. Cherkassky was organizer and Director of NATO
Advanced Study Institute (ASI) From Statistics to Neural
Networks: Theory and Pattern Recognition Applications held
in France in 1993. He received the IBM Faculty Partnership
Award in 1996 and 1997 for his work on learning methods for
data mining. In 2007, he became Fellow of IEEE for
‘contributions and leadership in statistical learning’. In
2008, he received the A. Richard Newton Breakthrough Award
from Microsoft Research for ‘development of new
methodologies for predictive learning’.
His current research interests
include methodological aspects of predictive learning and
advanced/non-standard formalizations for inductive
inference.
Optimized Assistive Human-robot Interaction Using Reinforcement Learning
F. L. Lewis, National Academy of Inventors. Fellow IEEE,
InstMC, IFAC
Moncrief-O’Donnell Endowed Chair and
Head, Advanced Controls & Sensors Group
UTA
Research Institute (UTARI), The University of Texas at
Arlington, USA
Qian Ren Thousand Talents
Consulting Professor, Northeastern University, Shenyang,
China
Personal website:
http://www.uta.edu/utari/acs.
Email:
lewis@uta.edu
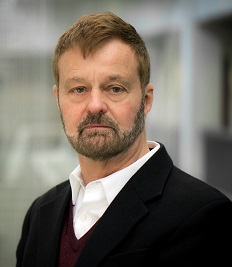
Abstract: Co-robotics involves humans and robots
working together safely in the same shared space as a team.
This motivates physical Human-Robot Interaction (HRI)
systems that adapt to different humans and have guaranteed
robustness and stability properties. For modern interactive
HRI systems to be capable of performing a wide range of
tasks successfully, it is required to include the effects
of both the robot dynamics and the human operator dynamics.
In this talk we propose three adaptive HRI control systems
that assist the human operator to perform a given task with
minimum human workload demands and improved overall
human-robot system performance.
Human performance
neuropsychological and human factors studies have shown
that in coordinated motion with a robot, human learning
has two components. The operator learns a robot-specific
inverse dynamics model to compensate for the
nonlinearities of the robot, and simultaneously learns a
feedback control component that is specific to the
successful performance of the task. These foundations can
be incorporated in the design of HRI control systems that
include the effects of both the robot dynamics and the
human dynamics by using a 2-loop design procedure.
In this talk, we develop an
adaptive HRI control structure consisting of two control
loops. First, a robot-specific neuro-adaptive controller
is designed in the inner loop to make the unknown
nonlinear robot behave like a prescribed robot impedance
model as perceived by a human operator. In contrast to
most existing neural network and adaptive impedance based
control methods, no information of the task performance
(e.g. specifically no reference trajectory information)
is required in the inner loop. Then, a task-specific
outer-loop controller is designed to find the best
parameters of the prescribed robot impedance model to
adjust the robot’s dynamics to the operator’s skills to
effectively perform a given task. The outer loop includes
the human operator dynamics and all the task performance
details. Given the inner-loop neuro-adaptive robot
controller, three different outer loop designs are given
for robot-assisted task performance. Experimental results
on a PR2 robot demonstrate the effectiveness of this
approach in using the robot to improve the human’s
performance of a motion task.
Biosketch F.L. Lewis:Member, National Academy of Inventors. Fellow IEEE, Fellow IFAC, Fellow U.K. Institute of Measurement & Control, PE Texas, U.K. Chartered Engineer. UTA Distinguished Scholar Professor, UTA Distinguished Teaching Professor, and Moncrief-O’Donnell Chair at The University of Texas at Arlington Research Institute. Qian Ren Thousand Talents Consulting Professor, Northeastern University, Shenyang, China. IEEE Control Systems Society Distinguished Lecturer. Bachelor's Degree in Physics/EE and MSEE at Rice University, MS in Aeronautical Engineering at Univ. W. Florida, Ph.D. at Ga. Tech. He works in feedback control, reinforcement learning, intelligent systems, and distributed control systems. He is author of 6 U.S. patents, 316 journal papers, 406 conference papers, 20 books, 48 chapters, and 12 journal special issues. He received the Fulbright Research Award, NSF Research Initiation Grant, ASEE Terman Award, Int. Neural Network Soc. Gabor Award 2009, U.K. Inst. Measurement & Control Honeywell Field Engineering Medal 2009. Received IEEE Computational Intelligence Society Neural Networks Pioneer Award 2012. Distinguished Foreign Scholar at Nanjing Univ. Science & Technology. Project 111 Professor at Northeastern University, China. Distinguished Foreign Scholar at Chongqing Univ. China. Received Outstanding Service Award from Dallas IEEE Section, selected as Engineer of the Year by Ft. Worth IEEE Section. Listed in Ft. Worth Business Press Top 200 Leaders in Manufacturing. Received the 2010 IEEE Region 5 Outstanding Engineering Educator Award and the 2010 UTA Graduate Dean’s Excellence in Doctoral Mentoring Award. Elected to UTA Academy of Distinguished Teachers 2012. Texas Regents Outstanding Teaching Award 2013. He served on the NAE Committee on Space Station in 1995.
Consensus Clustering Paradigms
Asoke K. Nandi, FREng, FIEEE, FIET, FIMA, FInstP, FRSA,
FIMechE, FBCS
Head of Department of Electronic
and Computer Engineering
Brunel University
London, Uxbridge, UB8 3PH, United Kingdom
Personal website: http://www.brunel.ac.uk/people/asoke-k.-nandi
Email: Asoke.Nandi@brunel.ac.uk
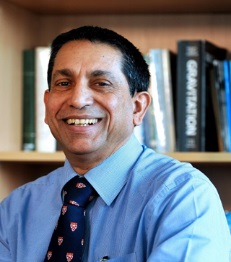
Abstract: Clustering techniques have been developed
and applied in many areas for several decades. In
particular, they have been used for gene clustering over
the last two or three decades in bioinformatics and brain
signal processing. New algorithms are being developed and
applied to address many different problems. However, in
applications with real data with little a priori
knowledge, it is often difficult to select an appropriate
clustering algorithm and evaluate the quality of
clustering results due to the unknown ground truth. It is
also the case that conclusions based on only one specific
algorithm might be biased, since each algorithm has its
own assumptions of the structure of the data, which might
not correspond to the real data.
Another important issue relates
to multiple datasets, which may have been generated
either in the same laboratory or different laboratories
at different times and with different settings yet trying
to conduct the similar experiments. In such a scenario,
one has essentially a selection of heterogeneous datasets
on similar experiments. The challenge is how to reach
consensus conclusions in such scenarios.
This plenary presentation will
address both of the aforementioned issues and discuss
how Bi-CoPaM and UNCLES can solve both the issues. This
presentation will include examples of results from some
bioinformatics and brain signal processing, although
these can be applied to all applications areas involving
clustering.
Bio-Sketch: Professor Asoke K. Nandi received the
degree of Ph.D. in Physics from the University of
Cambridge (Trinity College), Cambridge (UK). He held
academic positions in several universities, including
Oxford (UK), Imperial College London (UK), Strathclyde
(UK), and Liverpool (UK) as well as Finland Distinguished
Professorship in Jyvaskyla (Finland). In 2013 he moved to
Brunel University (UK), to become the Chair and Head of
Electronic and Computer Engineering. Professor Nandi is a
Distinguished Visiting Professor at Tongji University
(China) and an Adjunct Professor at University of Calgary
(Canada).
In 1983
Professor Nandi contributed to the discovery of the three
fundamental particles known as W+,W- and Z0 (by the UA1
team at CERN), providing the evidence for the unification
of the electromagnetic and weak forces, which was
recognized by the Nobel Committee for Physics in 1984.
His current research interests lie in the areas of signal
processing and machine learning, with applications to
communications, gene expression data, functional magnetic
resonance data, and biomedical data. He has made many
fundamental theoretical and algorithmic contributions to
many aspects of signal processing and machine learning.
He has much expertise in “Big Data”, dealing with
heterogeneous data, and extracting information from
multiple datasets obtained in different laboratories and
different times. He has authored over 500 technical
publications, including 200 journal papers as well as
four books, entitled Automatic Modulation Classification:
Principles, Algorithms and Applications (Wiley, 2015),
Integrative Cluster Analysis in Bioinformatics (Wiley,
2015), Automatic Modulation Recognition of Communications
Signals (Springer, 1996), and Blind Estimation Using
Higher-Order Statistics (Springer, 1999),. Recently he
published in Blood, BMC Bioinformatics, IEEE TWC,
NeuroImage, PLOS ONE, Royal Society Interface, and
Signal Processing. The h-index of
his publications is 63 (Google Scholar).
Professor Nandi is a Fellow of the Royal Academy of
Engineering and also a Fellow of seven other
institutions including the IEEE and the IET. Among the
many awards he received are the Institute of Electrical
and Electronics Engineers (USA) Heinrich Hertz Award in
2012, the Glory of Bengal Award for his outstanding
achievements in scientific research in 2010, the Water
Arbitration Prize of the Institution of Mechanical
Engineers (UK) in 1999, and the Mountbatten Premium,
Division Award of the Electronics and Communications
Division, of the Institution of Electrical Engineers
(UK) in 1998.
Deep Neural Networks for Supervised Speech Separation
DeLiang Wang
The Ohio State University, USA
Personal website:http://www.cse.ohio-state.edu/~dwang/
Email: dwang@cse.ohio-state.edu
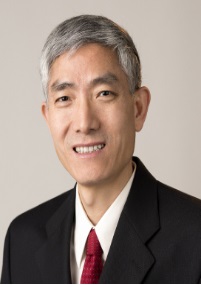
Abstract:Speech separation, or the cocktail party
problem, is a widely acknowledged challenge in speech and
signal processing. Motivated by the auditory masking
phenomenon, we have suggested the ideal binary mask (IBM)
as a main goal for speech separation. This leads to a new
formulation of the separation problem as supervised
classification where time-frequency (T-F) units are
classified into two classes: those dominated by the
target speech and the rest. This formulation opens speech
separation to modern machine learning techniques, and
deep neural networks (DNN) are particularly well-suited
for this problem due to their strong representational
capacity. DNN-based IBM estimation elevates speech
separation performance to a new level, and produces the
first demonstration of substantial speech intelligibility
improvements for both hearing-impaired and normal-hearing
listeners in background noise. DNN-based separation is
not limited to binary masking, and we have examined a
number of training targets and found that ratio masking
can be preferable in terms of speech quality, and T-F
masking in general outperforms spectral mapping.
Brief Biography: DeLiang Wang received the B.S.
degree and the M.S. degree from Peking (Beijing)
University and the Ph.D. degree in 1991 from the
University of Southern California all in computer
science. Since 1991, he has been with the Department of
Computer Science & Engineering and the Center for
Cognitive and Brain Sciences at The Ohio State
University, where he is a Professor. He also holds a
visiting appointment at the Center of Intelligent
Acoustics and Immersive Communications, Northwestern
Polytechnical University. He has been a visiting scholar
to Harvard University, Oticon A/S (Denmark), and Starkey
Hearing Technologies. Wang's research interests include
machine perception and neurodynamics. He received the
Office of Naval Research Young Investigator Award in
1996, the 2005 Outstanding Paper Award from IEEE
Transactions on Neural Networks, and the 2008 Helmholtz
Award from the International Neural Network Society. He
was named the University Distinguished Scholar by Ohio
State University in 2014. He is an IEEE Fellow, and
currently serves as Co-Editor-in-Chief of Neural
Networks.
Development of New Biometrics Applications
David Zhang, BSc (Peking), MSc. PhD (Harbin IT), PhD
(Waterloo), FIEEE, FIAPR
Department of
Computing, Hong Kong Polytechnic University, Hong Kong
Personal website:http://www.comp.polyu.edu.hk/~csdzhang
Email: csdzhang@comp.polyu.edu.hk
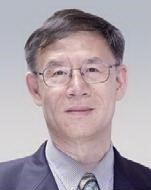
Abstract:As one of the most powerful and reliable
means of personal authentication, biometrics has been an
area of particular interest. It has led to the extensive
study of biometrics technologies and the development of
numerous algorithms, applications, and systems, which
could be defined as Advanced Biometrics. This
presentation will be focused on this new biometrics
research trend. As case studies, two new biometrics
applications (medical biometrics and aesthetical
biometrics) are developed. Some useful achievements
could be given to illustrate their effectiveness.
Bio-Sketch:David Zhang graduated in Computer
Science from Peking University. He received his MSc in
1982 and his PhD in 1985 in Computer Science from the
Harbin Institute of Technology (HIT), respectively. From
1986 to 1988 he was a Postdoctoral Fellow at Tsinghua
University and then an Associate Professor at the
Academia Sinica, Beijing. In 1994 he received his second
PhD in Electrical and Computer Engineering from the
University of Waterloo, Ontario, Canada. He is a Chair
Professor since 2005 at the Hong Kong Polytechnic
University where he is the Founding Director of the
Biometrics Research Centre (UGC/CRC) supported by the
Hong Kong SAR Government in 1998. He also serves as
Visiting Chair Professor in Tsinghua University, and
Adjunct Professor in Peking University, Shanghai Jiao
Tong University, HIT, and the University of Waterloo. He
is Founder and Editor-in-Chief, International Journal of
Image and Graphics (IJIG); Founder and and Series
Editor, Springer International Series on Biometrics
(KISB); Organizer, the 1st International Conference on
Biometrics Authentication (ICBA); Associate Editor of
more than ten international journals including IEEE
Transactions and so on. So far, he has published over 10
monographs, 400 journal papers and 35 patents from
USA/Japan/HK/China. According to Google Scholar, his
papers have got over 34,000 citations and H-index is 85.
He was listed as a Highly Cited Researcher in
Engineering by Thomson Reuters in 2014 and in 2015,
respectively. Professor Zhang is a Croucher Senior
Research Fellow, Distinguished Speaker of the IEEE
Computer Society, and a Fellow of both IEEE and IAPR.